In today’s fast-paced world, the insurance industry is undergoing a seismic shift. At the heart of this transformation lies the how of digital and analytics in insurance, a study point that’s reshaping the landscape for insurers and policyholders alike.
This comprehensive guide will navigate you through the intricate web of digital transformation and data analytics in the insurance sector, providing valuable insights for professionals and enthusiasts in the USA market.
Digital Transformation in Insurance
The winds of change are blowing strong in the insurance industry, with digital transformation at the helm. This shift isn’t just about adopting new technologies; it’s a fundamental reimagining of how insurance companies operate and deliver value to their customers.
The Digital Imperative
Insurance companies are facing unprecedented pressure to evolve. Here’s why:
- Customer Expectations: Today’s tech-savvy consumers demand seamless, personalized experiences across all touchpoints. They expect instant quotes, easy policy management, and quick claim settlements – all at their fingertips.
- Competitive Pressure: New entrants, including InsurTech startups, are disrupting the market with innovative, tech-driven solutions. These agile competitors are forcing traditional insurers to rethink their business models.
- Operational Efficiency: Digital tools offer the promise of streamlined processes and reduced costs. Automation can significantly cut down on manual tasks, freeing up human resources for more complex, value-adding activities.
- Regulatory Compliance: With ever-evolving regulations, digital solutions can help insurers stay compliant more easily and adapt quickly to changes.
Areas of Impact
Digital transformation is touching every aspect of the insurance value chain:
Area | Impact | Examples |
Customer Acquisition and Retention | AI-powered chatbots, personalized marketing | 24/7 customer service, tailored policy recommendations |
Underwriting and Risk Assessment | Machine learning models for accurate risk pricing | Real-time risk assessment, dynamic pricing |
Claims Processing | IoT devices for real-time claim reporting, blockchain for transparency | Automated claims settlement, fraud detection |
Product Development | Data-driven insights for tailored product offerings | Usage-based insurance, micro-insurance products |
Case Study: Progressive Insurance
Progressive Insurance has been at the forefront of digital innovation in the USA. Their usage-based insurance program, Snapshot, uses IoT devices to collect driving data and offer personalized rates. This approach has not only improved risk assessment but also enhanced customer engagement and loyalty.
“The companies that will thrive in the coming years will be those that can leverage digital tools to create more value for their customers.” – Tricia Griffith, CEO of Progressive
Progressive’s success with Snapshot demonstrates how digital transformation can create win-win situations for both insurers and policyholders. Customers benefit from potentially lower rates and increased awareness of their driving habits, while Progressive gains a more accurate risk assessment and improved customer retention.
Role of Analytics in Insurance
Data analytics is the engine driving the digital transformation in insurance. It’s turning vast amounts of data into actionable insights, enabling insurers to make smarter decisions and offer more value to their customers.
The Analytics Advantage
- Enhanced Decision-Making: From underwriting to claims, analytics provides a data-backed foundation for critical decisions. For instance, predictive models can help underwriters assess risk more accurately, leading to better pricing decisions.
- Personalized Customer Experiences: By analyzing customer data, insurers can tailor their offerings and communications. This could mean recommending the most suitable policy based on a customer’s life stage or sending targeted loss prevention tips.
- Fraud Detection and Prevention: Advanced analytics can spot suspicious patterns that might indicate fraudulent activity. Machine learning algorithms can analyze thousands of data points in real-time, flagging potentially fraudulent claims for further investigation.
- Improved Risk Assessment and Pricing: Predictive models can more accurately assess risk, leading to fairer pricing. For example, telematics data from cars can provide a more nuanced view of a driver’s risk profile than traditional factors like age and gender alone.
- Product Innovation: Analytics can uncover unmet needs in the market, driving the development of new insurance products. For instance, analysis of weather patterns and property data could lead to more tailored flood insurance offerings.
Challenges in Implementation
While the benefits are clear, implementing analytics in insurance isn’t without its hurdles:
- Data Quality and Integration: Insurers often struggle with siloed, inconsistent data. Legacy systems may not play well with newer data sources, making it challenging to get a holistic view of the business.
- Regulatory Compliance: Strict data privacy laws like GDPR and CCPA pose challenges. Insurers must balance the desire for data-driven insights with the need to protect customer privacy and comply with regulations.
- Talent Gap: There’s a shortage of professionals who understand both insurance and data science. This makes it difficult for insurers to build and maintain robust analytics capabilities.
- Cultural Resistance: Traditional insurance companies may face resistance from employees who are used to making decisions based on experience rather than data-driven insights.
Types of Analytics
Analytics in insurance isn’t one-size-fits-all. Let’s explore the spectrum of analytics and how each type contributes to the insurance ecosystem.
Descriptive Analytics
Descriptive analytics answers the question, “What happened?” It’s the foundation of data analytics in insurance.
Applications:
- Claims frequency and severity analysis
- Customer segmentation
- Performance tracking and reporting
Tools and Techniques:
- Data visualization software (e.g., Tableau, Power BI)
- Statistical analysis (e.g., mean, median, mode)
- Data mining
Descriptive analytics can help insurers understand trends in claims, identify their most profitable customer segments, and track key performance indicators over time. For example, an insurer might use descriptive analytics to create a dashboard showing claims trends by region, helping them allocate resources more effectively.
Diagnostic Analytics
Diagnostic analytics digs deeper, answering “Why did it happen?” This type of analytics is crucial for understanding the root causes of insurance outcomes.
Use Cases:
- Root cause analysis of claim spikes
- Identifying factors affecting customer churn
- Assessing the impact of marketing campaigns
Methods:
- Drill-down analysis
- Data discovery
- Correlations and regression analysis
Diagnostic analytics can help insurers understand why certain events occur. For instance, if an insurer notices a spike in auto claims in a particular area, diagnostic analytics could reveal that it’s due to a combination of bad weather and increased traffic due to construction work.
Predictive Analytics
Predictive analytics looks to the future, answering “What might happen?” It’s a game-changer in risk assessment and customer behavior prediction.
Applications:
- Risk scoring and premium pricing
- Claims prediction and reserve estimation
- Customer lifetime value prediction
- Fraud propensity models
Technologies:
- Machine learning algorithms (e.g., random forests, neural networks)
- Time series analysis
- Natural language processing for unstructured data
Predictive analytics can revolutionize how insurers assess risk and price policies. For example, a life insurer could use predictive models that incorporate data from wearable devices to more accurately assess an individual’s health risk and offer personalized premiums.
Prescriptive Analytics
The most advanced form of analytics, prescriptive analytics, answers “What should we do?” It provides recommendations for action based on analytical insights.
Real-World Examples:
- Optimizing policy offerings based on customer segments
- Automated underwriting decisions
- Proactive risk mitigation strategies
Challenges:
- Complexity of implementation
- Need for high-quality, real-time data
- Balancing automated decisions with human judgment
Prescriptive analytics can guide insurers on the best course of action. For instance, it could recommend the optimal mix of policy features for different customer segments or suggest personalized risk mitigation strategies for high-risk policyholders.
Emerging Technologies in Insurance Analytics
As the field of analytics evolves, new technologies are emerging that promise to further transform the insurance industry:
Artificial Intelligence and Machine Learning
AI and ML are becoming increasingly sophisticated, enabling more accurate predictions and automating complex decision-making processes. For example, AI-powered chatbots can handle routine customer inquiries, while machine learning algorithms can continuously improve fraud detection models.
Internet of Things (IoT)
IoT devices are providing insurers with unprecedented access to real-time data. In auto insurance, telematics devices can track driving behavior, while in home insurance, smart home devices can alert homeowners to potential risks like water leaks or fire hazards.
Blockchain Technology
Blockchain has the potential to streamline insurance processes and increase transparency. It could enable smart contracts that automatically execute policy terms, or create a shared ledger of claims data to prevent fraud.
Big Data and Cloud Computing
The ability to process and analyze vast amounts of data in the cloud is opening up new possibilities for insurance analytics. Insurers can now incorporate a wider range of data sources, from social media to satellite imagery, to gain a more comprehensive view of risk.
Case Study: Lemonade Insurance
Lemonade, a digital-native insurance company, has made waves in the industry with its AI-driven approach. Their chatbot, AI Jim, can handle the entire claims process in seconds for simple cases. This has led to unprecedented efficiency – Lemonade holds the world record for the fastest claim payment at just 3 seconds.
“By replacing brokers and bureaucracy with bots and machine learning, Lemonade promises zero paperwork and instant everything.” – Daniel Schreiber, CEO of Lemonade
Lemonade’s success illustrates how embracing digital technologies and advanced analytics can create a more efficient, customer-friendly insurance experience.
The Future of Insurance Analytics
As we look to the future, several trends are likely to shape the evolution of insurance analytics:
- Hyper-personalization: As data sources become more diverse and analytics more sophisticated, insurers will be able to offer increasingly personalized products and services.
- Real-time risk assessment: The combination of IoT devices and advanced analytics will enable insurers to assess and price risk in real-time, potentially leading to “on-demand” insurance products.
- Predictive prevention: Analytics will increasingly be used not just to predict risk, but to prevent losses. For example, insurers might use weather data and property information to alert homeowners to take action before a storm hits.
- Ecosystem integration: Insurance analytics will likely become more integrated with broader ecosystems. For instance, car insurance could be seamlessly integrated with smart city infrastructure to provide real-time risk information.
- Ethical AI: As AI becomes more prevalent in insurance decisions, ensuring fairness and transparency in algorithms will be crucial. Insurers will need to navigate the ethical implications of using AI in areas like pricing and claims decisions.
Conclusion
In conclusion, the digital and analytics revolution in insurance is not just reshaping the industry—it’s redefining it. From AI-driven underwriting to IoT-enabled risk assessment, the possibilities are boundless. The insurers who will thrive in this new landscape are those who can effectively harness the power of data and technology to deliver more value to their customers.
However, this journey is not without its challenges. Data quality, regulatory compliance, and the need for specialized talent are hurdles that must be overcome. Yet, the potential rewards—enhanced decision-making, personalized customer experiences, and improved operational efficiency—make these challenges worth tackling.
As we look to the future, one thing is clear: the how of digital and analytics in insurance will be the key differentiator in a highly competitive market. For insurers, the time to act is now. Embrace the digital revolution, invest in analytics capabilities, and prepare to lead in the new era of data-driven insurance.
FAQ’s
How does analytics improve insurance pricing?
Analytics improves insurance pricing by leveraging vast amounts of data to assess risk more accurately. It allows insurers to consider a wider range of factors, leading to more personalized and fair pricing. For example, telematics data in auto insurance can provide insights into individual driving behaviors, resulting in premiums that better reflect actual risk.
Can analytics help prevent insurance fraud?
Yes, analytics is a powerful tool in preventing insurance fraud. Machine learning algorithms can analyze patterns across thousands of claims, identifying anomalies that might indicate fraudulent activity. Real-time analytics can flag suspicious claims for immediate investigation, while predictive models can assess the likelihood of fraud before a claim is even filed.
How does predictive analytics benefit insurance customers?
Predictive analytics benefits customers by enabling more personalized insurance products and services. It can lead to fairer pricing based on individual risk profiles, faster claims processing, and proactive risk management advice. For instance, predictive models might suggest home maintenance actions to prevent potential claims, saving customers money and hassle in the long run.
What’s the role of IoT in insurance analytics?
IoT devices play a crucial role in insurance analytics by providing real-time, granular data. In home insurance, smart devices can detect water leaks or fire hazards, potentially preventing claims. In health insurance, wearables can track activity levels and vital signs, allowing for more accurate health risk assessment. This data enables insurers to offer more personalized policies and incentivize risk-reducing behaviors.
How is AI changing insurance analytics?
AI is revolutionizing insurance analytics by enabling more sophisticated data analysis and automation. Machine learning algorithms can process vast amounts of structured and unstructured data, uncovering insights humans might miss. AI-powered chatbots can handle routine customer inquiries and claims, improving efficiency. Natural language processing can analyze text data from social media or claim reports, providing additional context for risk assessment and fraud detection.
Also Read : The Power of 314159u in Driving Innovation and Growth
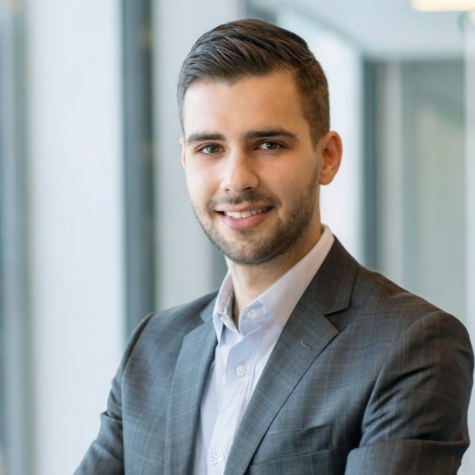